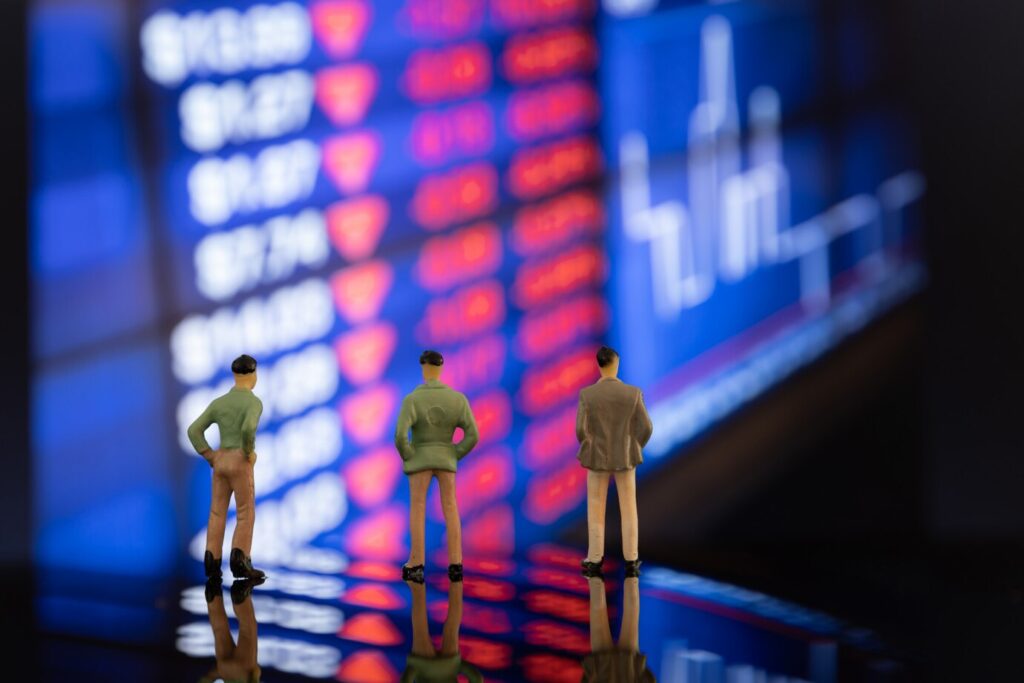
Can AI really predict stock market?
The stock market, by its very nature, is inherently unpredictable. However, with the advancement of AI, the possibility of using these powerful algorithms to forecast future market movements has become a captivating topic. This article delves into the capability of AI in predicting the stock market, exploring the limitations of current technology and the ongoing quest for reliable market forecasting solutions.
Understanding AI Algorithms
In the realm of stock market prediction, the significance of AI algorithms cannot be overstated. These sophisticated systems operate on the bedrock of Machine Learning (ML), a field within AI that empowers computers to learn from data.
At its core, ML enables these algorithms to sift through vast datasets, discern patterns, and make predictions based on historical and real-time information. The ability to process immense amounts of financial data swiftly sets AI apart as a formidable tool for market analysis.
In the context of stock markets, AI algorithms play a pivotal role in deciphering complex market trends. Their capacity to analyze and interpret intricate data sets gives them a unique edge in identifying patterns that might elude human analysts. This nuanced understanding of market dynamics positions AI as a valuable asset in navigating the complexities of stock trading.
In essence, the fusion of AI and financial markets hinges on the intricate algorithms that power these systems. Understanding the basics of Machine Learning and recognizing the role AI plays in scrutinizing market trends lays the groundwork for comprehending the ongoing debate on whether AI can genuinely predict stock market movements.
Success Stories
The marriage of Artificial Intelligence (AI) and stock market predictions has witnessed remarkable success stories, illustrating the transformative impact of these advanced technologies.
Precision in Market Downturns
One notable success lies in AI’s ability to accurately forecast market downturns. Advanced algorithms have demonstrated an impressive capacity to recognize subtle indicators and provide timely warnings, allowing investors to navigate volatile periods with foresight.
Timely Decision-Making
AI-driven predictions have empowered investors with the capability to make timely decisions. By swiftly processing and analyzing vast datasets, these algorithms provide insights that go beyond human capacity, ensuring that investors can capitalize on fleeting opportunities or shield themselves from potential losses.
Strategic Investment Planning
In the realm of strategic investment planning, AI has showcased its prowess. Success stories abound where AI algorithms have identified undervalued stocks, optimized portfolios, and devised investment strategies that outperform traditional methods. These instances highlight the potential for AI to not only predict market movements but also to enhance overall investment strategies.
These success stories underscore the tangible benefits AI brings to the table, reinforcing the notion that, when wielded effectively, AI has the potential to revolutionize the landscape of stock market predictions.
Challenges and Limitations
To navigate the nuanced landscape of AI-driven stock market predictions, it’s crucial to recognize the challenges and limitations associated with these advanced algorithms. Let’s delve into a comprehensive overview, breaking down the key aspects through a structured table and subsequent detailed points:
Challenges | Limitations | Impact |
Overfitting | Data Quality | Market Volatility |
Model Complexity | Lack of Interpretability | Uncertain Economic Factors |
Changing Dynamics | Ethical Concerns | Regulatory Compliance |
Overfitting:
- Challenge: Overfitting occurs when an AI model learns to perform exceptionally well on historical data but struggles to generalize to new, unseen data. This phenomenon poses a significant challenge in the context of stock market predictions, as markets evolve, and historical patterns may not always repeat.
- Impact: Overfit models can lead to inaccurate predictions, potentially resulting in financial losses for investors who rely solely on historical data for decision-making.
Model Complexity:
- Challenge: The complexity of AI models can be a double-edged sword. While intricate models can capture subtle market nuances, they may also become less interpretable, making it challenging to understand the rationale behind specific predictions.
- Impact: Investors and analysts may hesitate to trust or implement predictions from overly complex models, leading to a lack of adoption in the financial industry.
Changing Dynamics:
- Challenge: Financial markets are dynamic, influenced by a myriad of factors, including geopolitical events, economic shifts, and sudden market sentiment changes. AI models may struggle to adapt quickly to these changing dynamics.
- Impact: Failure to adapt swiftly can result in outdated predictions, rendering AI less effective in navigating rapidly evolving market conditions.
In summary, while AI holds immense potential in stock market predictions, understanding and mitigating these challenges and limitations is crucial for fostering trust and maximizing the effectiveness of these advanced technologies.
Improving Predictive Models
Enhancing the accuracy and reliability of predictive models in stock market analysis is an ongoing pursuit. Here are key strategies to improve these models:
Continuous Learning Algorithms
- Dynamic Updating:
- Implement algorithms that can dynamically update based on real-time market data.
- This ensures models remain relevant in the face of evolving market conditions.
- Adaptive Parameters:
- Utilize models with adaptive parameters, allowing them to adjust to changing patterns.
- This flexibility enhances the model’s ability to capture emerging trends.
- Ensemble Learning:
- Embrace ensemble learning techniques that combine multiple models.
- This approach leverages the strengths of diverse models, mitigating individual model weaknesses.
Integration of Diverse Data Sources
- Alternative Data Sets:
- Incorporate alternative data sets beyond traditional financial data.
- Social media sentiment, satellite imagery, and other non-conventional sources offer valuable insights.
- Big Data Analytics:
- Harness the power of big data analytics to process and analyze vast datasets efficiently.
- This includes leveraging cloud computing resources for scalable and timely analysis.
- Cross-Validation Techniques:
- Employ cross-validation to assess model performance across various data subsets.
- This helps identify potential biases and ensures the model’s generalization to diverse scenarios.
By adopting these strategies, the predictive models can evolve, becoming more robust and adaptable to the ever-changing landscape of the stock market. Continuous learning algorithms and the integration of diverse data sources contribute to the development of models that are not only accurate but also resilient in the face of uncertainties.