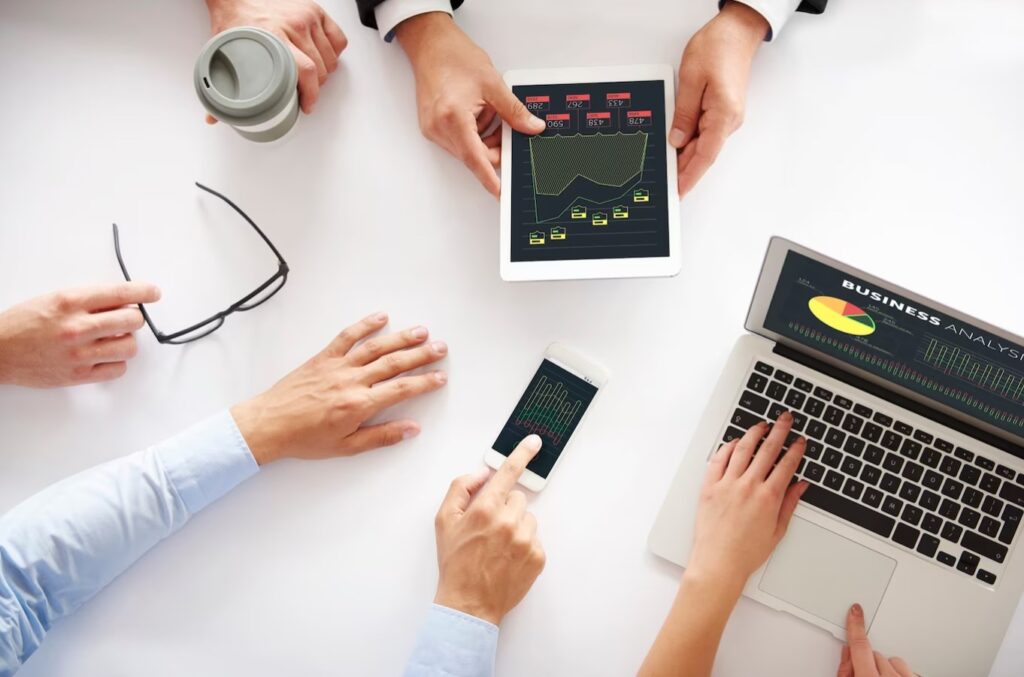
Do banks use AI for trading?
In the fast-paced world of finance, staying ahead of the curve is paramount. Banks, as major players in the market, are constantly exploring innovative solutions to optimize their operations. This article investigates the prevalence of AI adoption in bank trading practices, analyzing the potential benefits and challenges associated with integrating this technology into their financial strategies.
The Evolution of AI in Banking
The journey of Artificial Intelligence (AI) in banking has been marked by a fascinating evolution. Initially, during the early stages of computerization, the financial industry embraced technology to automate routine tasks and enhance data processing capabilities. This laid the groundwork for more sophisticated applications of AI.
As technology advanced, particularly in recent decades, banks recognized the need for more intelligent systems to cope with the complexities of modern finance. This recognition led to the integration of AI in various facets of banking operations, including customer service, fraud detection, and, significantly, trading.
The emergence of AI in trading represents a pivotal moment in the evolution of banking technology. With the increasing speed and complexity of financial markets, traditional methods of trading became insufficient. In response, banks turned to AI to optimize trading strategies. This shift led to the development of algorithmic trading, where powerful algorithms execute trades at high speeds, capitalizing on market opportunities in ways that human traders couldn’t match.
In summary, the evolution of AI in banking reflects a dynamic interplay between technological advancements and the ever-changing demands of the financial landscape. From the early stages of computerization to the integration of sophisticated AI-driven trading strategies, the trajectory highlights the industry’s commitment to leveraging cutting-edge technology for enhanced efficiency and decision-making.
How Banks Utilize AI for Trading
Algorithmic trading represents a paradigm shift in the way banks execute trades. Utilizing sophisticated algorithms, banks can process vast amounts of market data in real-time. These algorithms analyze price movements, trading volumes, and other relevant indicators to identify patterns and trends. The beauty of algorithmic trading lies in its speed and precision – it can execute trades at a pace impossible for human traders. This approach not only maximizes the potential for profit but also minimizes the impact of emotional decision-making, a common pitfall in traditional trading.
Predictive Analytics
In the realm of trading, predictive analytics powered by AI is a game-changer. Banks leverage predictive models that utilize machine learning algorithms to analyze historical data and identify potential future market trends. By understanding past patterns and behaviors, these models make predictions about the likely direction of prices, enabling banks to make more informed trading decisions. This data-driven approach enhances the accuracy of forecasting and empowers banks to anticipate market movements, giving them a competitive edge in the fast-paced world of financial markets.
In summary, the utilization of AI in trading extends beyond just executing transactions. Algorithmic trading optimizes the execution process, while predictive analytics enables banks to foresee market trends, combining to create a powerful strategy for navigating the complexities of the financial landscape.
Advantages of AI in Banking
Artificial Intelligence (AI) has ushered in a new era for banks, offering a multitude of advantages that significantly impact various aspects of their operations. Here’s a closer look at the key benefits:
- Improved Efficiency:
- Streamlined Processes: AI automates repetitive tasks, allowing banks to streamline their operations and allocate resources more efficiently.
- Faster Decision-Making: The speed of AI-driven systems enables quicker analysis of data, leading to faster decision-making processes.
- Enhanced Decision-Making:
- Data-Driven Insights: AI processes vast datasets to provide valuable insights, empowering banks to make informed decisions based on comprehensive information.
- Risk Assessment: AI’s ability to analyze risk factors contributes to more accurate risk assessments, aiding in prudent decision-making.
- Mitigation of Risks:
- Fraud Detection: AI algorithms excel in detecting unusual patterns and anomalies, enhancing banks’ capabilities to identify and prevent fraudulent activities.
- Compliance Management: AI ensures adherence to regulatory requirements by continuously monitoring transactions and activities for compliance.
In essence, the advantages of AI in banking extend beyond operational enhancements. They touch upon efficiency, decision-making prowess, and risk management, collectively contributing to a more robust and resilient financial ecosystem.
Challenges and Concerns
Challenges | Concerns | Mitigation Strategies |
Ethical Considerations | Data Privacy Concerns | Implementation of Ethical Guidelines |
Technological Complexity | Bias in AI Algorithms | Regular Audits and Algorithm Transparency |
Integration Hurdles | Security Risks | Robust Cybersecurity Measures |
- Ethical Considerations: With the increasing reliance on AI, banks must navigate ethical considerations surrounding issues such as data privacy, fairness, and accountability.
- Technological Complexity: The complexity of AI systems poses challenges in terms of implementation, integration with existing infrastructure, and the need for specialized expertise.
- Integration Hurdles: Integrating AI solutions into existing banking systems and processes can be challenging, requiring careful planning and resource allocation.
In summary, while AI offers immense potential for banks, it also presents a range of challenges and concerns that must be addressed to ensure its responsible and effective implementation. By proactively addressing these challenges and adopting mitigation strategies, banks can harness the full benefits of AI while mitigating associated risks.
Real-world Examples
In the realm of banking, real-world applications of Artificial Intelligence (AI) in trading have demonstrated remarkable success. Several financial institutions have embraced AI-driven technologies to enhance their trading strategies and overall operational efficiency.
One compelling example is JPMorgan Chase, a global banking giant. JPMorgan has implemented AI algorithms for algorithmic trading, enabling rapid and precise execution of trades. The algorithms analyze market data in real-time, identifying patterns and opportunities that human traders might overlook. This implementation has not only optimized trading processes but has also contributed to improved profitability for the bank.
Another noteworthy case is Goldman Sachs, which employs AI for predictive analytics in trading. The investment bank utilizes machine learning algorithms to analyze historical market data, identify trends, and predict potential price movements. This predictive capability enhances Goldman Sachs’ ability to make informed trading decisions, ultimately gaining a competitive edge in the dynamic financial markets.
Additionally, Morgan Stanley stands out for its innovative use of AI in risk management. The bank leverages AI algorithms to assess and mitigate various risks associated with trading activities. The technology’s ability to process vast datasets in real-time enables Morgan Stanley to respond swiftly to changing market conditions, ensuring a robust risk management framework.
The Future of AI in Banking
The trajectory of Artificial Intelligence (AI) in banking points towards exciting developments and innovations. Here are key aspects shaping the future of AI in the financial sector:
- Advanced Machine Learning Algorithms:
- Embrace more sophisticated algorithms for enhanced pattern recognition and predictive analytics in trading and risk management.
- Explore deep learning models to uncover intricate market patterns and improve decision-making processes.
- Expanded Use of Natural Language Processing (NLP):
- Facilitate improved customer interactions through advanced chatbots and virtual assistants.
- Utilize NLP for in-depth analysis of unstructured data, extracting valuable market insights and sentiments.
- Increased Personalization in Customer Services:
- Harness AI algorithms to analyze customer behavior and preferences.
- Offer personalized financial solutions and recommendations, enhancing overall customer experiences.
- Blockchain Integration for Enhanced Security:
- Integrate AI with blockchain technology to bolster security measures in banking operations.
- Leverage AI-powered algorithms for robust fraud detection and secure transactions on decentralized platforms.
- Continued Growth of Robotic Process Automation (RPA):
- Increase adoption of RPA for automating routine tasks, reducing operational costs, and improving overall efficiency.
- Deploy AI-driven robots for tasks ranging from data entry to compliance checks, freeing up human resources for more complex functions.
- Augmented Analytics for Decision Support:
- Implement augmented analytics, combining AI and data analytics, to provide comprehensive decision support.
- Enable decision-makers to derive actionable insights from vast datasets, facilitating more informed and strategic choices.
The future of AI in banking holds immense promise, with these trends poised to revolutionize how financial institutions operate, making processes more efficient, secure, and customer-centric.