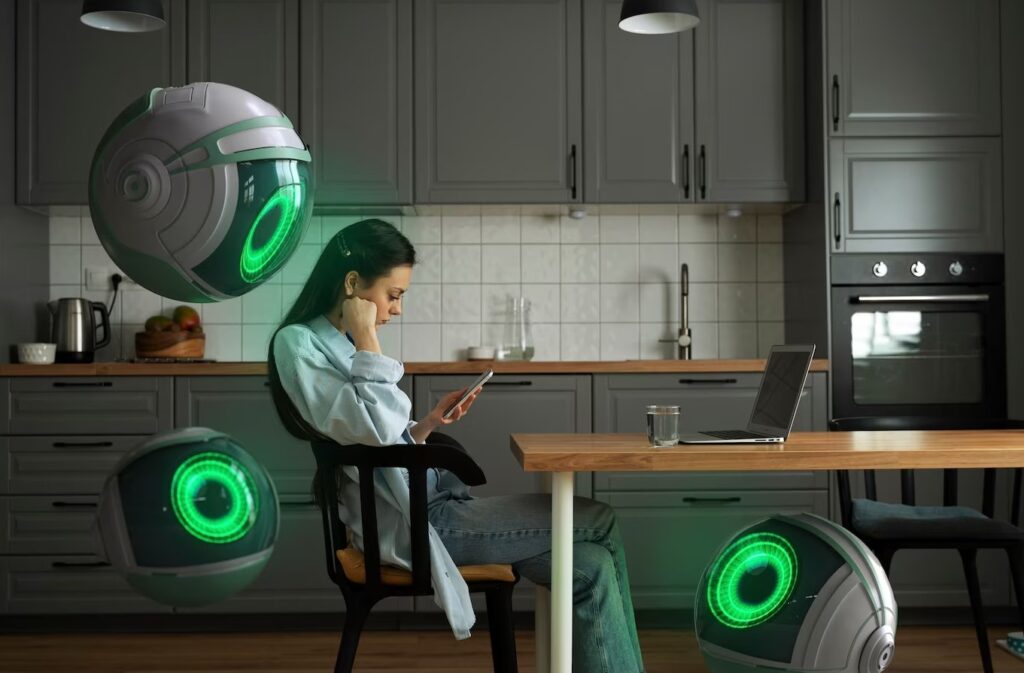
What percent of traders are bots?
The world of finance is rapidly evolving, and with it, the role of human traders. The rise of automation has sparked curiosity about the balance between human intuition and algorithmic precision. This article delves into the intriguing question: what percentage of trades are actually executed by bots? We’ll explore the current landscape of algorithmic trading and its impact on traditional human involvement in the financial markets.
The Rise of Algorithmic Trading
Over the years, the landscape of trading has undergone a remarkable transformation. Traditional methods have given way to the era of algorithmic trading, marking a significant paradigm shift in the financial markets.
Algorithmic trading, often referred to as algo-trading, involves the use of computer programs to execute trading strategies at a speed and frequency that surpass human capabilities. This evolution stems from the need for faster and more efficient trade execution in an increasingly dynamic market environment.
The introduction of algorithmic trading has been propelled by advancements in technology, allowing for complex algorithms to analyze vast amounts of market data and execute trades with precision. This shift has not only revolutionized the speed of transactions but has also brought forth a range of advantages and challenges for market participants.
Traders now have the ability to execute orders at speeds measured in microseconds, responding to market changes in real-time. The efficiency and accuracy of algorithmic trading have contributed to improved market liquidity and reduced bid-ask spreads, benefitting both institutional and retail investors.
However, this surge in algorithmic trading has not been without its drawbacks. The automation of trades has led to concerns regarding market manipulation, as algorithms can execute large volumes of trades in a fraction of a second, influencing prices and triggering market fluctuations.
Quantifying Bot Presence
Understanding the percentage of traders that are bots poses a unique challenge due to the dynamic and decentralized nature of financial markets. Quantifying bot presence involves navigating through various complexities and relying on studies and research to shed light on this intricate landscape.
Challenges in Determining Bot Percentage
- Dynamic Nature of Trading Environment: Financial markets are constantly evolving, making it challenging to capture an accurate snapshot of bot presence at any given moment.
- Masking Techniques: Sophisticated trading algorithms can employ masking techniques, making it difficult to distinguish bot-driven activities from human-initiated trades.
- Decentralized Exchanges: With the rise of decentralized exchanges, tracking and quantifying bot activity become even more challenging, as there is no central authority overseeing trading activities.
Studies and Research on Bot Prevalence
- Academic Studies: Academic institutions conduct research to analyze trading patterns and identify algorithmic behavior, contributing valuable insights into the prevalence of trading bots.
- Market Surveillance Tools: The use of advanced market surveillance tools by exchanges and regulatory bodies enables the identification of abnormal trading patterns, indicating potential bot activities.
- Collaborative Efforts: Collaboration between industry experts, exchanges, and regulatory bodies fosters a collective approach to quantifying bot presence, utilizing shared resources and expertise.
The Role of Exchanges in Bot Regulation
- Implementing Controls: Exchanges play a pivotal role in implementing controls to identify and regulate bot activities, imposing restrictions and safeguards to maintain market integrity.
- Transparency Initiatives: Some exchanges have taken proactive steps to enhance transparency, providing regular reports on bot activities and ensuring traders are well-informed about the prevalence of algorithmic trading.
- Continuous Monitoring: Continuous monitoring of trading activities by exchanges, coupled with real-time reporting mechanisms, contributes to the ongoing effort to quantify and regulate bot presence in financial markets.
In navigating the complexities of quantifying bot presence, it is evident that a multi-faceted approach involving technological advancements, collaborative research, and proactive regulatory measures is essential. As we delve deeper into this intricate landscape, the quest to determine the percentage of traders that are bots requires ongoing vigilance and innovation to maintain the integrity of financial markets.
Factors Influencing Bot Adoption
To comprehend the factors influencing the adoption of trading bots, it’s essential to navigate a landscape shaped by technological advancements, market conditions, and regulatory dynamics. Here’s a breakdown of the key influences, presented in a table for clarity:
Factors | Description | Impact on Bot Adoption |
Technological Advancements | Rapid developments in algorithmic strategies and AI. | Accelerates the adoption as traders seek efficiency. |
Market Conditions | Volatility, liquidity, and speed of market changes. | Flourishes in high-frequency trading during volatility. |
Regulatory Landscape | Legal frameworks governing algorithmic trading. | Adoption influenced by compliance and regulatory clarity. |
Technological Advancements
- Algorithmic Strategies: Ongoing advancements in algorithmic strategies, including machine learning and artificial intelligence, drive the adoption of sophisticated trading bots.
- Data Processing Speed: Faster data processing capabilities enhance the efficiency of trading bots, making them more appealing to traders seeking quick execution.
- Integration with APIs: Seamless integration with application programming interfaces (APIs) allows bots to access real-time market data and execute trades swiftly.
Market Conditions
- Volatility Opportunities: Trading bots thrive in volatile markets, where rapid price movements create opportunities for quick and precise trades.
- Liquidity Considerations: Bots are attracted to liquid markets, as higher liquidity allows for smoother execution of trades without significant price slippage.
- Speed of Market Changes: The ability of trading bots to react instantaneously to changes in the market landscape positions them as valuable assets in fast-paced trading environments.
Regulatory Landscape
- Compliance Requirements: Traders consider the legal implications of using bots, with adherence to compliance requirements influencing the decision to adopt algorithmic trading.
- Regulatory Clarity: Clear and well-defined regulatory frameworks provide a sense of security, fostering a conducive environment for the widespread adoption of trading bots.
- Risk Mitigation Measures: Traders assess how regulatory guidelines address the risks associated with algorithmic trading, influencing their confidence in adopting bot technologies.
Understanding these factors and their interplay is crucial in grasping the trajectory of bot adoption in financial markets. The intricate balance between technological evolution, market dynamics, and regulatory frameworks shapes the landscape for traders considering the integration of algorithmic solutions.
Identifying Bot Activity
Identifying bot activity in the complex landscape of financial markets requires a nuanced approach and the utilization of specialized tools. Traders and regulatory bodies employ various methods to distinguish between automated algorithmic trading and human-initiated actions.
Common Characteristics of Bot-Driven Trades
- High-Frequency Trading Patterns: Bots often exhibit high-frequency trading patterns, executing a large number of orders within a short time frame.
- Consistent Reaction Time: Unlike human traders, bots react consistently and rapidly to market changes, showcasing a uniform reaction time.
- Large Order Sizes: Bot-driven trades may involve large order sizes, aiming to capitalize on price movements and market inefficiencies.
Tools and Techniques for Detecting Bots
- Market Surveillance Software: Specialized market surveillance software is designed to analyze trading patterns and detect anomalies indicative of bot activity.
- Order Book Analysis: Monitoring the order book allows for the identification of unusual order placements and rapid order cancellations, common traits of algorithmic trading.
- Algorithmic Trading Indicators: Traders use specific indicators designed to spot algorithmic trading strategies, such as statistical arbitrage or trend-following algorithms.
- Behavioral Analysis: Examining trading behavior over time helps identify consistent patterns, enabling the differentiation between human and bot-driven actions.
- Machine Learning Models: Advanced machine learning models are employed to recognize complex patterns in trading data, enhancing the ability to identify and classify bot activity.
- Collaborative Information Sharing: Traders and regulatory bodies collaborate to share information and insights, creating a network effect that enhances the collective ability to identify and understand bot-driven trading.
Understanding the common characteristics of bot-driven trades and deploying sophisticated tools for detection are critical components of maintaining market integrity. As technology advances, so do the methods employed to identify and regulate algorithmic trading activities, ensuring a fair and transparent trading environment for all participants.
Impacts on Traditional Trading
The impact of algorithmic trading on traditional trading practices is profound, reshaping the dynamics of financial markets in ways that both benefit and challenge traditional traders. One notable effect is seen in market liquidity and volatility. Algorithmic trading contributes to increased liquidity, making it easier for traders to buy or sell assets. However, this enhanced liquidity also introduces a level of market volatility as algorithmic strategies respond rapidly to market changes, leading to swift price fluctuations.
Traditional traders face new challenges as algorithmic trading dominates certain market segments. The speed at which bots execute trades often surpasses human capabilities, making it challenging for traditional traders to compete on equal footing. This has prompted a shift in trading strategies, with traditional traders adapting to coexist with algorithmic counterparts or exploring alternative markets where human expertise remains a decisive factor.